
The Correlation Between Dark Web Exposure and Cybersecurity Risk
We are joined by the Marsh McLenan Cyber Risk Intelligence Center to discuss a landmark study that has quantified the impact of dark web exposure for the first time.
Can you quantify the risk the dark web poses to organizations?
In this episode of the podcast we discuss a landmark study that has tried to do just that.
We’re joined by Scott Stransky, Managing Director and Head of the Marsh McLennan Cyber Risk Intelligence Center and Ben Jones, CEO of Searchlight Cyber and Scott unravel the findings of the report “The Correlation Between Dark Web Exposure and Cybersecurity Risk”.
Click here to download the research report discussed in the podcast: https://slcyber.io/whitepapers-reports/the-correlation-between-dark-web-exposure-and-cybersecurity-risk/
Apply for a Dark Web Risk Report on your organization.
Speakers

Ben Jones
Co-Founder and CEO of Searchlight Cyber
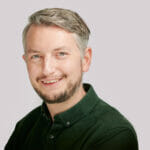
Aidan Murphy
Host

Scott Stransky
Managing Director and Head of the Marsh McLennan Cyber Risk Intelligence Center
In this episode of The Dark Dive we discuss:
How cyber insurance loss data can be used to calculate the impact of dark web exposure on an organization's cybersecurity risk
Marsh McLennan correlated Searchlight's dark web intelligence against cyber insurance data on 9,410 organizations.
How different types of dark web exposure individually impact the chance of a cyberattack
Including Dark Web Market Listings, Mentions in Dark Web Forums, and Dark Web Traffic to and from the organization.
How multiple factors combined increase the chances of a cybersecurity incident
Marsh McLennan's multi-variable analysis shows that visibility of multiple areas of dark web exposure leads to a more reliable estimate of risk.
Transcript
Aidan Murphy: Hello, and welcome to another episode of The Dark Dive, the podcast that delves into the depths of the dark web. My name is Aidan Murphy, and I’m your host as each month, we look at a different aspect of the dark web. In this episode, we’re going to look at how the dark web relates to cybersecurity risk. Regular listeners to the podcast will have heard a...
Aidan Murphy: Hello, and welcome to another episode of The Dark Dive, the podcast that delves into the depths of the dark web. My name is Aidan Murphy, and I’m your host as each month, we look at a different aspect of the dark web. In this episode, we’re going to look at how the dark web relates to cybersecurity risk. Regular listeners to the podcast will have heard a lot about cyber criminals targeting companies from the dark web in all manner of ways. We’ve talked about data exfiltrators selling huge stolen data sets on dark web marketplaces, initial access brokers auctioning vulnerabilities on dark web forums, and of course, ransomware groups blackmailing victims on the dark web leak sites. This stands to reason then that the dark web holds inherent risk for organizations, but can that risk be quantified? On this episode of the podcast, we’re going to discuss a study, launched in September 2024, that has tried to do just that. Joining me to discuss this research are representatives of the two organizations behind the study, Scott Stransky, managing director and head of the Marsh McLennan Cyber Risk Intelligence Center. Hello, Scott.
(TC: 00:01:04)
Scott Stransky: Hello.
(TC: 00:01:05)
Aidan Murphy: And Ben Jones, CEO and co-founder of Searchlight Cyber. Hello, Ben.
(TC: 00:01:09)
Ben Jones: Hi.
(TC: 00:01:09)
Aidan Murphy: So, before we get into the detail of the report, I’m just going to ask each of you to introduce yourself to the listeners. Scott, if it’s alright, I’m going to start with you. Could you give us a little bit of background on yourself and the Marsh McLennan Cyber Risk Intelligence Center? Which, from this point onwards, I’m just going to refer to as Marsh McLennan for brevity’s sake, but please, Scott, yes, introduce yourself and the center.
(TC: 00:01:30)
Scott Stransky: Yes, so I’m Scott Stransky, I lead what we call the Marsh McLennan Cyber Risk Intelligence Center. Our goal is really to quantify cyber risk using every data source possible. We have a lot of our own proprietary data sources, which I’ll talk about throughout this podcast, including insurance loss data. For those who aren’t familiar with Marsh McLennan, we are one of the world’s largest risk advisors. One of our main functions is as an insurance broker, meaning we help companies acquire and place insurance, and because of that, we get a lot of information about these companies risk profiles. Things like, well, do they have an insurance claim? These are going to come to us to help them manage that claims process, and this is very important data that we can use in our risk quantification, and it was used for the study we’ll talk about here today. Other functions of our center includes evaluating third-party data sets, such as your own, and then thought leadership, including working with academic institutions, really all to advance the state of cyber risk quantification and cyber risk analytics, and I’m sure we’ll get into a lot more details of this as we go through the podcast.
(TC: 00:02:31)
Aidan Murphy: Brilliant. Thanks, Scott, that was a great overview and yes, lots of stuff I’m going to come back to. Ben, so regular listeners will have heard of Ben before. Ben was in an episode in season one, but Ben, just for the new listeners who might be joining us for the first time, maybe you could introduce yourself and your role as CEO of Searchlight Cyber.
(TC: 00:02:49)
Ben Jones: Hi, yes, so my name is Ben Jones, I’m the CEO and co-founder of Searchlight Cyber. Gareth and I, our CTO, set up the company to help protect society against the threats of the dark web, and the work that we’ve done here is an extension of that, helping to protect companies and those companies that hold government and personal information, as well as protecting their own infrastructure. So, this is an extension of that mission in terms of protecting society from those threats.
(TC: 00:03:18)
Aidan Murphy: Brilliant, thank you, Ben, and we’re going to have a lot of conversation, as always, about the dark web. So, thank you both for joining me, and we’re here to really discuss a joint report between Marsh McLennan and Searchlight Cyber, which is titled, I should say, The Correlation Between Dark Web Exposure and Cybersecurity Risk. I’m going to start at the beginning. So, Scott, what prompted you to, and I guess Marsh McLennan, to investigate dark web intelligence as a source in the first place.
(TC: 00:03:46)
Scott Stransky: So, there are a lot of reasons why we wanted to look into this type of data. First of all, we had looked at other types of data over the past few years. We’ve, kind of, had a theme each year of the data sources we look at. I started in this role about three years ago, and the first data source that we looked at was what we call outside-in data. These are scans that are done of companies unobtrusively from the outside, and we found some correlation between those scores that are found from the scans and loss data. Now, this is great, it’s useful in our cyber risk quantification models, but it’s done by basically the entire insurance industry. It’s nothing exceptionally novel and unique. It’s important to quantify for sure, but it’s not something that would really advance the state of the cyber quantification. So, coming into the second and third years of our center, we wanted to look for a new data source that was used by cybersecurity professionals perhaps, but wasn’t necessarily in practice in the insurance industry for helping evaluate cyber insurance risk, and dark web seen liked the obvious place for a few reasons. One is it scales. If you think about say, inside-out data, which is also very interesting to us, it’s tough to scale, because you need a company’s permission to get that data, so you can’t get it for thousands or tens of thousands of companies at once. On the other hand, with dark web data, you can get it on any company who sits on the dark web, so working with vendors like yourselves, we were able to understand, for 10,000 companies, what was going on in the dark web, and this was a very appealing thing for this type of data.
So, we decided let’s evaluate dark web data. We have a very structured process for evaluating data sets like this. We have a portfolio, a set of 12,000 or so companies, that we share with anybody who wants to participate in a vendor study. These are not 12,000 random companies, these are 12,000 companies that we have a very good idea whether they’ve have had a cyber insurance loss or not. If you just think about cyber insurance in general, if you’ve purchased a cyber insurance policy and you suffer an incident, you’re probably going to want to collect on your cyber insurance, or else why would you be paying for that cyber insurance in the first place? So, while there are lots of breach databases, incident databases out there, they have biases. They’re useful, don’t get me wrong, they have a lot of use, but looking at insurance data gives us that unbiased view. It sure is a smaller universe, but it’s really the only way to get this unbiased view. So, it’s a worldwide data set, and we shared this with a bunch of different dark web vendors, and we had them basically give us everything they knew about these companies. They could be counts, it could be yes or nos, like, did they find something or not? It could be multiple choice things, lots of different ways that they could have potentially approached giving us data, and it could be as many fields as they wanted to. We got this data back from multiple vendors, and the good news is they were all correlated in the right direction, meaning more things that were found in the dark web leads to more incidents, more cyber insurance losses.
That’s something you would expect, and we’ll talk a lot more about the details of that with your data as we move through the podcast, but just at a high level, all the data sources we looked at were correlated. You’ll not I use the word correlated throughout this, and I’m using that word very very carefully. Our studies are looking for correlation, meaning an association between things that we found on the dark web, and more or fewer cyber insurance losses. That’s different than using the word prediction. I know some people casually use the word prediction. We don’t like to use that. We’re not able to do an experiment here, we can’t make a company, like, have the bad actors talk about a company and then not talk about a different company and see which one gets breached. This is not an experiment, which is why I’m specifically using the word correlation, which is important. To find a correlation means that we can use this data within our cyber risk quantification process, which is used across our organization to help customers understand how much cyber insurance they need to buy, whether there are cost benefits to improving their cyber security and more. So, that’s a very high level overview of why we wanted to do this study, to try to find correlation between dark web data, such as searchlights, and cyber insurance loss data, which we believe is an unbiased view of insurance and cyber risk in general.
(TC: 00:07:45)
Aidan Murphy: That’s a great overview, Scott. Sorry, I just want to come back on a couple of things you mentioned there. So, when you say loss data, effectively, these are organizations that have had an incident and then made a claim, that’s how we know that something has happened, right?
(TC: 00:08:00)
Scott Stransky: Yes, our hypothesis is that if you’ve spent the money to get cyber insurance, which we think is a very good thing to do, and you do suffer an incident, you’re going to want to tell us as your cyber insurance broker, about that information. You may not want to publicize it, which is why it may not make the news, it may not make articles online, which is why the publicly reported data sets, which again, they have value. They don’t have as much value for this type of study, simply because they’re biased. They’re biased towards say publicly traded companies that have an obligation to report, biased towards bigger companies, which may have customer data, and if that gets stolen they have to tell people about it. On the other hand, cyber insurance, even if you’re a small business, and you don’t have to report the breach publicly, if you have cyber insurance, you’re probably going to tell your insurance broker. We keep it very confidential. I’ll just tell everybody here, Searchlight Cyber didn’t see any of the claims data that we have. We can’t share with anybody. We can’t share it with any vendor that we work with, ever. All of the analysis and data work was done in-house, on my team, which is for everybody’s protection. I don’t want people to get oh, I was breached, I told my-, no, no, no, no. It’s very very sensitive data, we get that. We were able to do all the analysis on our side.
(TC: 00:09:05)
Aidan Murphy: Yes, that’s a very important point to make obviously, but it’s a good summary of why this data is so valuable, like you say, people are going to make a claim if they have experienced loss, and you said you looked at other dark web intelligence vendors as well, and all of them were correlated. Was that unusual? Was that something you expected, or does that speak to dark we intelligence being a particularly good source for, well, correlation to claims?
(TC: 00:09:35)
Scott Stransky: So, we try to go into these studies unbiased, without really a hypothesis. I know, like, in the scientific method you should go in with a hypothesis that this data is going to be correlated, but we actually try to go in with an open mind and let the data drive the direction. We basically say, ‘Give us any data you want to give us and we’ll see if it’s correlated.’ We don’t contrive that, we don’t say, ‘Oh, this better be correlated, let’s squeeze out the correlation.’ No, no, we don’t do anything like that. We get any data and vendor’s willing to give us, and we see if it’s correlated. We were very happy and pleased to see that all this data was correlated, but that’s not the impression we went in with. Obviously, I’m sure Searchlight knew in advance that the data was going to be correlated, but we did not, we purposely did not. We wanted to go in, sort of, blindly. We wanted to do a true blind study of the correlation.
(TC: 00:10:21)
Aidan Murphy: Well, I guess maybe we should ask Ben. So, I assume, Ben, going into this, you know, the assumption is, before we actually get into the results and what the results show, that if an organization has exposure on the dark web, and we can talk about some of those different exposures in a second, we would expect it to be positively correlated to increase cybersecurity risk.
(TC: 00:10:42)
Ben Jones: We would, yes, and this is one of the reasons why we’ve started moving into this style of security and providing that outside in approach to your network. Originally, we started off around working with law enforcement to try and help identify and protect against criminal activity against citizens as a whole, but we did find the data that we had gathered and some of the intelligence that we put together, there are indicators of compromise within a company’s infrastructure, with the information that we found. So, whether that’s around traffic data, or people looking to sell access to that network, this is why we produced the DarkIQ product because of those things which we had seen, and that there is value to be had there, and it does help organizations protect their networks from that type of activity. So, any information that will give you some pre-warning about something that’s going to happen to your network is very valuable.
(TC: 00:11:40)
Aidan Murphy: Yes, and I thought it was quite interesting the way Scott described it earlier, because we also sometimes talk about this, kind of, outside-in approach, so you can see the threat on the outside and they relate to, like you say, compromises that may have taken place, or be about to take place, on the inside.
(TC: 00:11:56)
Scott Stransky: Yes, I think that’s a huge advantage from our perspective because we’re going to work with our clients and they may not want to install something behind their firewall, they may not want to let us inside of their network. I know they own this dark web data, similar to the outside and scanning data. You can get it on them without really their permission or their knowledge, you can just get it, so that’s very appealing for us when we’re thinking about building models for that type of risk.
(TC: 00:12:18)
Aidan Murphy: Brilliant, so Scott, I’m going to stick with you for a second. So, can we talk about the methods? So, you looked at other vendors, Searchlight Cyber was selected to run this study, and then what was the methodology behind the study then? So, you mentioned I think 12,000 organizations, but in the end, it’s 9,410, if I remember correctly off the top of my head that we have in the study.
(TC: 00:12:40)
Scott Stransky: Yes, so we wanted to make sure that we weren’t double counting anything. We wanted to make sure that we had proper data on all the companies. Yes, it ended up being about 9,410 companies in the study. Again, these are companies going back maybe four or five years or so, where we’re know if they’ve had a claim or not, and what we ask you all to do was give us your data fields, again, open-ended, you can give us whatever you want, on these companies, going back four or five years. We wanted to make sure we weren’t biasing it by something that happened after a cybersecurity incident, meaning you gave us data, say from 2022, for breaches that happened in 2022. We didn’t want data from 2024 for breaches that happened a couple of years ago. That was actually quite important to us, because we feel like companies may fix things after a breach, or in some cases, they may deteriorate even more. We didn’t want to have to deal with that issue, which is why it was important for us to get that historical data as we’re thinking about who’s been breached or not. So, we were able to get data on all of these companies, the 9,410, going back through about 2020 or so, and then what we did was we joined your data to our data set before the breach happened, as I suggested. Now, again, we didn’t know what you gave us, in fact you could have given us one of the fields as truly a random number. Some of our vendors do that to try to test us or trick us. We’re fine with that. It turns out you didn’t do that, but that’s fine, we’re open to that, and then we did a correlation study.
So, we looked at what is the probability that you had a breach, given that something was found on the dark web, versus what is the probability that you had a breach, given that nothing was found on the dark web. You’ll note I carefully use something and nothing found on the dark web. We actually studied the amount of things found on the dark web as well. We found that wasn’t quite as correlated as simply something or nothing, which that was maybe counter-intuitive to us. We thought if you had one versus ten versus 1,000 versus 10,000 exposed things in the dark web, it would make more of a difference. It turns out going from zero to one makes a huge difference, and going from one to other numbers doesn’t make nearly as much of a difference. It was still correlated, but we found the most correlation, actually by far, just going from zero to one. That’s effectively how we did the study, and then we built confidence intervals for those who like statistics, or if you didn’t like statistics, you can cover your ears for the next few moments. Basically, we built 95% confidence intervals around those point estimates, meaning probabilities you had a breach given we found something, probabilities you had a breach given we didn’t find something, and the good news is that there was no overlap between those confidence intervals, meaning if you found some on the dark web, you always had an elevated risk of having a cyber incident, compared to if you didn’t find something on the dark web. Again, this was very exciting to us. All the nine fields that you all sent us had that signal, meaning finding something was leading to more cyber insurance losses than not finding something.
(TC: 00:15:26)
Aidan Murphy: I’m very happy you decided to tackle the confidence intervals, Scott, because it’s something I struggle to get my head around, but I think you very succinctly summarized it there, so that’s really good.
(TC: 00:15:36)
Scott Stransky: So, basically, it means that we’re 95% confident that these results are correct. If you’ve ever seen polling for elections, I know here in the United States we’re going through an election now, there are always polls. They give some, sort of, margin of error in confidence around that. It’s the same idea as that. You can’t be 100% certain ever. If we were 100% certain about something, we would all be out of our jobs. Here we’re 95% certain, and we are 95% confident that this data, this data about dark web, for all nine of the fields we looked at from Searchlight, has correlation between the data and having a cyber insurance loss.
(TC: 00:16:09)
Aidan Murphy: Yes, and I will just say at this point, so we’re going to go into some of the findings now. If you’re interested in having a look yourself, and if you’re particularly interested in the confidence intervals, I would urge you to download the report, which you’ll find in the show notes and the appendix, there is, kind of, detail on those confidence intervals and the rigor of the study, which I would encourage people to look at, especially if they are interested in the statistics around it. I am very quickly just going to run through those nine fields, we’re going to go into these in more depth as well, but the nine fields that we provided from a cyber perspective were compromised users, dark web market listings, outgoing dark web traffic, ingoing dark web traffic, OSINT results, paste results, telegram chats, forum posts and dark web pages. I know that was a very very quick overview. Again, I would urge you to look at the report to go into more depth on those. We are going to be going into more depth now on the podcast, but just to make you aware what those nine dark web intelligence sources that we went out to find on these 9,000-plus companies were. So, Scott, there are two analyses in the report.
(TC: 00:17:12)
Scott Stransky: Yes, so there are two different types of analyses in the report. The first was what we call a single variable analysis. Here what we did was we took each of the nine fields independently and did this correlation exercise, so we treated one at a time, ignoring the other eight, and that’s where we found a significantly significant correlation for each. The second analysis we did is actually, in our view, the one that we’re going to be using in our modeling, and it’s the more fundamental approach that accounts for all the fields at once. So, we did a multi-variable analysis, and again, if you don’t like statistics, cover your ears just for a moment, but what we did was a logistic regression methodology for all nine of these fields. The reason that we had to do this is because there’s some correlation between the nine fields. So, for example, if you have something bad on the dark web in one of the nine, you’re more likely to have something bad in one of the other of the nine as well. So, we can’t just include all nine of these things in our model because it’s, again, it’s effectively double counting the impact of some of the fields. So, by building a logistic regression, it actually effectively squeezes out the most important factors from the analysis. It turns out that five of them are what end up showing up as very important within this logistic regression model. These five fields now are not as correlated to each other, so we can include them all within a model, we don’t have to worry about that double counting problem.
Again, there are a lot of statistics behind this, a lot of math behind it. I’m not going to get into all the math here, but from a practitioner’s perspective, looking at the single variable analysis shows that each field is great on its own, but if you want to combine them all into a risk profile, you can’t just multiply the impacts of each field by each other. Again, it would be double counting the impact of some of them because they’re correlated, which is why we had to do the multi-variable analysis. Hopefully, that’s at a high enough level. Again, I’m happy to get much more detailed with the statistics. If people want to reach out to me after this podcast who like numbers and math, happy to chat about the details of this study with anybody.
(TC: 00:19:01)
Aidan Murphy: Yes, that was a great review, Scott. So, I am going to go into both of those analyses in a little bit more depth, but, Ben, before I get there, I guess from either one of those analyses, or just the report in general, was there anything that stood out for you as, kind of, like, the one key take away for people? If listeners go away and read the report or not, is there something they should take away as the key findings from your perspective?
(TC: 00:19:24)
Ben Jones: Yes, I mean, I think one of these things that the report helps quantify is one of the areas, which the reason why we developed the DarkIQ product is that organizations should get visibility into their exposure on the dark web. We believe that there is a connection between the information that you find there and your chances of getting breached, and therefore, we would encourage everybody to include dark web sources within their threat intelligence, and then if they can use a product like DarkIQ to help make it actionable as well, that’s even better.
(TC: 00:20:01)
Aidan Murphy: Yes, because I guess the finding of the single variable analysis, so as you said, Scott, I think it’s really worth underlying this, all nine sources individually are correlated to increase cybersecurity risk, so I guess then, Ben, that means that if you have visibility of say four of the sources but the other five, there is a chance that you are exposed somewhere on the dark web, and there is inherent risk in there, because that’s what this report shows. All of these sources are correlated with risk, but you’re just completely unaware of it, which is in itself a danger.
(TC: 00:20:37)
Ben Jones: Yes, and it’s not a surprise that some of these data sources are linked as well. I mean, you would expect, because of the way that some of these sources work, you end up with a tiered system where some of the most valuable things are disclosed within more private forums or more private market listings, and then as they become older, they then get, sort of, packaged together and then resold. So, you would expect things to come up in multiple different places, but if you want to be able to stand the best chance of being able to protect yourself against these things, is it is important to make sure that you have all of these elements in there. So, you can’t just use one element and say, ‘Okay, well, they’re all correlated, therefore if I just use this one data source, I’m pretty much covered.’ I think another conclusion from this study is that’s not the case, and that goes along with what I said before, even though there is some timing that’s been built into this analysis, it’s not super high-resolution in that fact that if it was leaked three months before, or three days before, that I don’t think the analysis will pick that up at this point. However, you want to be able to get visibility over that as soon as possible because it then gives you the best chance to be able to react to it. So, by having multiple different data sources in there, it’s really important. If you just monitor the more easily available ones, then you’re less likely to get that early warning, which could make the difference between a huge breach and a sigh of relief.
(TC: 00:22:04)
Scott Stransky: Yes, and indeed to Ben’s point, we did a bunch of different studies with the data. We looked at the data in the twelve months leading up to the cyber insurance loss, which is what we used in the study, but we also looked at three months, six months, two years before. It turns out that the twelve-month period leading up to the cyber insurance loss had the highest correlation with an incident. Again, there could be various explanations for that, but that’s what the data indicated is that adding up the things that were found in the past twelve months, was the most correlated with incidents.
(TC: 00:22:33)
Ben Jones: And that does, sort of, chime with our experience as well, because this whole element is now an ecosystem. So, you don’t just have one person who will find a way into an organization and then level up with it and then steal the data and then run a ransom against them, that’s generally not how these things work. There’s a whole ecosystem, and people will specialize in different elements of that, and therefore, the original compromise, it may well have been twelve months before the actual breach, because they will spend some time researching and trying to escalate throughout an organization. So, getting visibility of that early, compromise all that early access into your networking, will give you a lot more time to be able to react, and this goes to my third point really, which is it’s important to continuously measure this. If you’re just doing an annual audit, that’s quite a low resolution to be watching out for this type of data. You need to be able to continuously monitor it, because the earlier you get this, the stronger chance you have of being able to do something about it, and so if you do continue this every day, or in a continuous way, and then act on the data that you receive, you stand a much better chance of being able to do something about it before it escalates into a huge problem.
(TC: 00:23:57)
Aidan Murphy: Yes, so I guess that comes to this point that what we’re looking at here is data that exists outside of the organization, so like you say, Ben, the initial compromise might be a year before, and just to illustrate this for listeners, so that might be a year before on a forum someone says they’ve managed to get access to a particular organization. Exactly as you say, Ben, that access might not be exploited for another year, but if you can see it at that point, you can take action then to close the vulnerability, or change the password, or whatever it is they’ve managed to compromise, which might mean the account never transpires.
(TC: 00:24:34)
Ben Jones: I mean, we’ve seen examples where cleaners, who have access to particular areas, realize they have access to areas of sensitivity, and they then put it out there to say, ‘Look, I’ve got access to this place, what do I do with this access? How do I make some money out of this? Or I’m disgruntled as an employee and this is my employer, how do I cause them harm?’ And then we’ve seen those sorts of conversations happening, and then people advise them what they can do, and they’ll realize that they can also make money out of that, and then suddenly they’re now part of that ecosystem and providing access into criminal actors who then want to go and exploit that for financial gain.
(TC: 00:25:11)
Aidan Murphy: Yes, absolutely. So, just sticking with the single variable analysis for a second then to give listeners some idea of the stats behind this, looking at the top three findings, I guess in this single variable analysis, so we found that compromised users was the top level of exposure, and, Scott, I’m going to lean on you to correct me when I want to use the wrong language here. So, we found that increased the likelihood of a cyber incident by 2.56 times, relative to if you had no finding.
(TC: 00:25:42)
Scott Stransky: That’s correct. I’ll actually make an interesting point about compromised users in particular. So, the overall breach rate in our set of organizations that we care about is around 4%, 4.5%, so that’s, kind of, the base line. So, I’ll say compromised users is a little bit different than some of the other ones, where almost all of the organizations were yes for that, not all of them, but the vast majority were yes for. So, it’s actually almost the opposite for that one. If you have a no, it quote, unquote, reduces your risk, as opposed to if you have a yes, it increases your risk from the base line. Some of them are like that, where fewer have yes, but this is one where most of the organizations have a yes, so it actually, quote, unquote, gives you a lower risk if you have a no there. Some of the other ones, for example, telegram chats, not nearly as many companies have telegram chats, so there the base line is no, and that increases your risk by having a yes. It’s a subtle difference, but as you look through the report, again, keep in mind the base line is around 4.5%, so whichever column is close to that 4.% is, sort of, the base line, whichever column is either much higher or lower than that, is the special version. I don’t know if special is the right word, but the interesting version.
If they’re both far away from the 4.5%, then it’s 50-50 whether the company has it or not, and it’s the same as what I was saying, it’s more like okay, yes or no makes that difference, but in the case-, specifically because you brought it up, that the compromised users, that one, again, I’m going to say over 80% of the companies we study have that, so first of all, you shouldn’t feel terribly bad if you have that, but if you don’t have that, you’re actually at a benefit, as opposed to the opposite, where the base line is you don’t have it, and it hurts you if you do. I don’t know if that makes sense, hopefully that makes sense.
(TC: 00:27:16)
Aidan Murphy: No, it does make sense. I mean, one way of looking at that I guess is that so, if you’re listening, if you can ensure that there are not-, so when we’re talking about compromised users, we’re talking about accounts, so passwords, user names that we have found out there on the dark web. If you can make sure that is not the case for your organization, I think what Scott is saying is that would put you actually in the minority of organizations, and mean that you have a reduced chance of making an insurance claim, which is-,
(TC: 00:27:45)
Scott Stransky: Yes, I’m going to say, like, less than 20% of companies had no for that, so that’s where the vast majority, the base line is you do have that, which is why it improves your security if you say no. Again, telegram channels is the opposite, where the vast majority said no, or had no telegram exposure, and if you do have it, it makes you worse.
(TC: 00:28:04)
Ben Jones: I think just goes to show how pervasive this is, and the reality is that most people will probably have some form of compromised users out there, so it’s another point, it doesn’t matter how big your organization is, there are many different ways in which you can compromise a user. So, it’s not necessarily an active pursuit in order to try and focus on a given organization. Quite often, a lot of these tactics are passive, and so it could be that you have a computer that has been compromised with malware, and they will then strip off all of your user accounts and passwords associated with that. So, you may well have an employee who’s using their company laptop or browser for doing personal things as well as doing the logging on to their work accounts, and so it’s not necessarily through things like phishing sites or spearfishing. It’s quite often through some of these more passive systems, where people have managed to either exfiltrate information off of your computer by using malware, or using things like breached data sets, where somebody that you do business with has been breached, and then your e-mail and password combination has been leaked that way, and if you have bad password hygiene and you use similar passwords for different sites, that could then compromise your as well. I mean, honestly, most people do repeat passwords, and it’s one of the things that you can do to try and protect yourself, and it’s not easy to remember lots of different passwords, but that is one way in which you can escalate from your Amazon account up to your work account, is if you’re using these same passwords.
I would suggest the speed at which you can react to these things, and also the strength of your password policies and things like 2FA, will make the difference between whether you end up becoming compromised or not, and sometimes this is just down to pure luck as well. It’s that somebody is not after your particular organization, and then the credentials expire before they become useful, but do you really want to leave something like a breach down to luck?
(TC: 00:30:15)
Scott Stransky: Yes, and that’s actually consistent with a study that we did on our own data, a couple of years back. One of the things that Marsh has is something called a cyber self-assessment, and this is a questionnaire that our clients fill out. It’s, sort of, an inside view as they’re trying to acquire insurance, and to be a multi-factor authentication, there are three different questions on there about MFA. One is about MFA for internal employees, one is about MFA for administrators, and one is about MFA for crown jewels, and what we found, again, correlation, is that if you say yes, you have it for all three of those, you have a significantly reduced chance of having a cyber insurance loss over the next year. If you say no to even one of those things, it doesn’t change your risk at all, meaning you really have to say yes, you do it for all three things to reduce your risk, but we’ve done lots of correlation studies, including with our own data, and that’s one of the things we found consistent with what you’ve just said.
(TC: 00:31:05)
Aidan Murphy: Yes, I think there are a lot of other studies out there as well that, I mean, consistently find compromised users, leaked credentials, to be still the main way of getting into organizations, so I think is very consistent with what people will understand in the market. The other two at the top might be slightly different actually, so after compromised users, we have dark web market listings, which increases your likelihood of a cyber incident if you have it versus if you don’t, by 2.41 times. So, Ben, maybe if we just expand on this one as well, so what are we talking about when we talk about dark web market listings in relation to an organization?
(TC: 00:31:46)
Ben Jones: So, this can cover everything from physical goods being sold under that brand through to digital assets, and so this is another route in which people can sell access on to your network. So, it may not be accessed via a user’s account, but it could also include access via a VPN, so this is a part of this ecosystem where potentially somebody has now gained access to your system, they’ve escalated it, and they’ve now managed to get remote access to a particular machine, and they’re looking at selling that remote access. It could also be things like intellectual property. If you’ve had intellectual property stolen, once again, you may have already been breached in order for them to be able to get that information, and therefore looking to sell that information going forwards, and so there are multiple things that it could be, and dark net markets cover everything from drugs, all the way through to digital assets, like getting access to your network via a remote access VPN or something like that.
(TC: 00:32:46)
Aidan Murphy: And if listeners are interested, we do have an episode in season one specifically about dark web marketplaces, so we’d recommend to go back and listen to that as well, and the third as well, we’ll stop on three, but just as a reminder, all nine were found to be correlated, so again, please check the report for the other ones, but the third is an interesting one as well, which is outgoing dark web traffic. Ben, we haven’t actually talked a huge amount about dark web traffic on the podcast, so this might require a little bit of an explainer for you. When we talk about dark web traffic in this sense, can you give listeners an idea of, you know, exactly what we’re talking about. Where is this traffic going to? Where is it going from? What does it signify?
(TC: 00:33:26)
Ben Jones: Yes, so in this particular instance, dark web, we mean Tor. Colloquially, dark web could mean many things, but it’s generally those hidden parts of the internet, and so in this particular case, we’re talking about Tor traffic, and this is traffic leaving the dark web and going to your organization, or coming from your organization and going to the dark web. To be able to monitor the traffic going back and forth is valuable, because if you have a lot of data going between your network and Tor, people quite often will use Tor as a way of exfiltrating data as an extra layer of security for them. So, the Tor traffic is encrypted and routed through multiple different hops, which means it’s impossible to track where it’s going through. So, if I was a criminal and I wanted to get access to your network and I wanted to exfiltrate data, Tor is a useful layer to have within that, sort of, operational security, is to be able to bring that information through without anybody being able to trace it. So, if you have large volumes of traffic coming in and out of your organization, that could well be somebody who’s trying to exfiltrate data out, or it could be somebody who’s looking for vulnerabilities within your system and trying to hide behind Tor and use it a veil so that you can’t work out who they are.
(TC: 00:34:55)
Aidan Murphy: So, that, kind of, explains why this has ranked quite highly then in terms of being correlated to the likelihood of a cyber incident, because like you say, Ben, if you have-, this is specifically outgoing dark web traffic, if you have traffic going from your network out to the dark web, that signifies something bad already underway. Like you say, that could be data exfiltration, it could perhaps be malware has already been installed and is beaconing out to the dark web. So, this is quite a solid indicator that something-, I mean, taking the study to aside, when we work with organizations and we discover they have dark web traffic leaving their organization and going to the dark web, we would say this is quite a solid indicator that there is something that needs to be investigated, there is something suspicious happening there.
(TC: 00:35:43)
Ben Jones: I mean, there’s only really one good reason why you would have Tor traffic coming from your network, and that’s down to the fact that you have security researchers within your organization who are maybe using Tor to do some research. Now, this is a rather risky thing to be doing. There is a lot of malware, for example, on Tor, so if you are going to go on Tor, you want to use something like the stealth browser that we have, where you can go on in a sandboxed environment and you can do your research and access Tor that way, and then when you shut down the instance, any malware or infections, anything like that, are then deleted with the end of your session. Other than that, the types of traffic coming from your network to the Tor, just, sort of, like, escalate in terms of risk, so it could be somebody just looking to bypass your firewall on their lunch break, for example, to be able to go out and do some internet shopping or stream some TV, through to somebody who is trying to deliberately get data out of your organization, and there are various different reasons why. It could also be that you have people using Tor on their mobile phones or something like that, as part of accessing your wi-fi network. Once again, do you really want those, sort of, activities going on within your network? I would suggest probably not, and so, yes, it makes sense that this one of the more correlating, more positive correlations, in terms of breach.
(TC: 00:37:07)
Aidan Murphy: Brilliant. So, moving on to the multi-variable analysis then, and, Scott, you’ve already given a really good explainer on why the multi-variable analysis is important, but I guess just to go back to it, so this, in you view, well, in everyone’s view probably, is a more accurate way of looking at the risk if more than one of these sources is present. Like you say, because there could be crossover between sources, this is a more accurate way of, kind of, taking out that overlap. Is that one way of explaining it?
(TC: 00:37:38)
Scott Stransky: Yes. So, what you can’t do is if you look at each of the nine factors, one is two point something, one is two point something, one is one point-, you can’t just multiply those together to get the overall impact of having all of them. It doesn’t work that way because there’s a lot of correlation between these things. If you have one thing bad, you probably have other things bad. So, that’s why we had to do the multi-variable analysis. We couldn’t build it into our modeling and our work otherwise. So, yes, the multi-variable analysis uses what we call logistic regression modeling, and if you don’t know statistics, I apologize, that may not mean much to you, but it’s a form of model that allows to account for each of the variables and interaction between the variables. When we do that, five of the features come out as important or significant. It doesn’t mean that the others are bad, and in fact, if you’re a security professional, you should be looking at all of them, but if you’re trying to say, ‘Okay, what is the impact of having these things?’ You can’t look at all of them because the correlation between them. So, that’s why these five things came out from the multi-variable analysis, and this is how we use the data in modeling in particular, as opposed to say from a practitioner’s perspective.
(TC: 00:38:44)
Aidan Murphy: Brilliant, that’s such a good description. So, yes, like you say, there were five that came out of this as being, like you say, significant in combination, so those were paste results, OSINT results, again, and then we have the three that were ranked quite highly in the single variable analysis, so dark web market listings, outgoing dark web traffic, and compromised users, which I guess speaks to, again, the value of those sources.
(TC: 00:39:10)
Scott Stransky: Yes, it doesn’t mean that the others are bad, it just means that there’s a strong correlation between say forum posts and credentials being stolen, or whatever it happens to be, and we just don’t want to double count that as we’re modeling things.
(TC: 00:39:22)
Aidan Murphy: Absolutely, but doing it this way does mean that you can calculate combined risk. Is that right, Scott?
(TC: 00:39:27)
Scott Stransky: Yes. That’s the advantage of doing the multi-variable modeling, because now you can take these factors that come out of this analysis, and now you can actually combine them into a combined view of risk that says your X percent more likely than your average company to have a cyber security incident, based on what we found on the dark web.
(TC: 00:39:44)
Aidan Murphy: Yes, so in the report, we have a few examples of this. So, again, I recommend downloading the report, which will explain it much clearer, but for example, compromised users will give you a 7% increased likelihood of suffering a cyber security incident relative to your peers. Dark web market listings would give you a 13% increased likelihood relative to your peers, but you can combine those two different elements for an overall combined risk of 21%.
(TC: 00:40:11)
Scott Stransky: Yes, I just want to chime in there because somebody may hear 7%, 13%, add seven and thirteen, you get twenty, yet you said 21, you’re right that it’s 21, it’s because you actually have to multiply seven is 1.07, the thirteen is 1.13, you multiply the 1.07 by the 1.13, that’s how you get the 1.21. Again, I just want to make sure people get the basic math of what’s going on here and aren’t confused by seven and thirteen equals 21.
(TC: 00:40:35)
Aidan Murphy: No, that’s absolutely a fair point. So, if you took all five of those factors, so for example, if you imagined an organization had paste results, OSINT results, dark web market listings, outgoing dark web traffic and compromised users, again, multiply all of those percentages together, they would then have a 77% combined risk.
(TC: 00:40:54)
Scott Stransky: Compared to their peers, yes.
(TC: 00:40:56)
Aidan Murphy: Compared to their peers. Again, if you would like to do the math yourselves, download the report, use your calculators, but we have run it through and it does add up, or multiply together, as it actually might be, but just to explain what we’re talking about when we’re talking about combined risk. Ben, I guess from our perspective, exactly as you said before, this completely makes sense, and I imagine often, when we look or work with organizations, they tend to have more than one of these factors, so this is for them, also a more accurate way of looking at their cybersecurity risk, relative to their dark web exposure.
(TC: 00:41:31)
Ben Jones: Yes, I agree. This is where you need to look at the multiple sources, and the more of those sources that you can see are the ones which have a strong correlation, the better chance you’ve got of catching this as well. So, there is a higher correlation with having a breach, but that also then gives you a greater insight into what you should be looking at and how you should be responding to that. So, you can use that as an increased correlation, but you can also use it in the way that the more of these things you look, the greater chance you’ve got of catching something before it turns into something major.
(TC: 00:42:03)
Aidan Murphy: Yes, and I think it’s an important point, because as both you and Scott have said, this is not to say that the other sources are not valuable, and I think it is important to highlight, so, you know, you look at these percentages and they look smaller than the 2.11 times we were talking about in the single variable analysis, but what we’re talking about here is a higher degree of accuracy and accounting to the fact that there is overlap.
(TC: 00:42:25)
Scott Stransky: And the other key thing to note, and it mentions this in the report, is that in this multi-variable analysis, maybe a subtle point, but it’s actually really important, we control for the revenue or the turnover and the industry of companies, because to build a model you need to do that. In the individual independent analysis, we didn’t control for anything like that, it was truly everything else equal. In this multi-variable analysis, it’s a much fairer analysis to the world because we’re controlling for things like revenue and industry. Bigger companies tend to be bigger targets in general, whereas smaller companies aren’t. Some industries are much bigger targets. Also, like, finance, retail, etc, whereas maybe mining and agriculture are not as big targets. So, the multi-variable analysis absolutely accounts for revenue and industry in a way that the individual one does not. Again, that’s why the numbers are-, yes, you see the 2.5 times in one and 77% in the other. There are different mathematical things going on behind the scenes in the multi-variable analysis.
(TC: 00:43:19)
Aidan Murphy: I guess they also correlate with-, industry and revenue also correlate with other factors as well. Is that right, Scott? So, for example, if you’re, you know, a large bank with hundreds of thousands of employees, you are probably more likely to have leaked passwords out on the dark web, just by-,
(TC: 00:43:34)
Scott Stransky: Absolutely, which is why the multi-variable analysis is, kind of, the most pure analysis that we can think about. Again, it’s not something that a CISO or practitioner can use as easily, but it’s something that a quantifier of risk like myself absolutely needs. If you want to build a financial model, I know there’s a 77% number. 77% of what is the next question that I would have. That’s where we come in, and we can actually quantify the dollars or pounds or euros lost based on this. So, we can say without this finding, here’s the risk, with the finding, here’s the risk, in terms of actual money, and that’s really the key. If you’re a big company, that number is going to be a lot higher than if you’re a small company, but that 77% still applies.
(TC: 00:44:13)
Aidan Murphy: So, I mean, Scott, I think that leads on really well. So, from your perspective, I guess if you think about your customer base, what should they be taking away from this? How do you envisage this research being carried forward and used?
(TC: 00:44:27)
Scott Stransky: Yes, I mean, all of our cyber insurance customers come to us and are getting analytics are lost quantifications. We are building this Searchlight data into our model, that’s going to be a key part of the model, along with the outside-in data we’ve talked about, some of the questionnaires we talked about, all of our proprietary data. The idea is that this will now influence the analytics we give to our clients. They’ll be able to see the impact of these things and financial losses, which will help them understand how much insurance they need to buy. Perhaps if they have not much going on in the dark web, we’d say, ‘Yes, save a little bit of money now. You’re doing a great job with your cybersecurity so you don’t need to invest this much in insurance.’ On the other hand, if we see a lot of problems, obviously you can help them rectify some of those problems, but we could also suggest maybe you need to buy a bit more insurance this year, there are some pretty sketchy things going on in the dark web about your company, you have an elevated risk. Not a guarantee that you’re going to have an incident, of course, but you have an elevated risk, and of course, we are the largest insurance broker, so we’re the place to come if you want to buy cyber insurance, and these companies can figure out the right amount of cyber insurance to buy using our models.
(TC: 00:45:29)
Aidan Murphy: Brilliant, and Ben, from our perspective, so if we’re talking down one side, but on the enterprise perspective, what should people take away from this? What should their next steps be? They’ve read this report, you know, they’ve seen conclusively dark web exposure relates to cybersecurity risk, what do they do next?
(TC: 00:45:47)
Ben Jones: Yes, so in terms of quantifying those risks from a financial point of view, obviously, we don’t know anything about that, but what we do know about is security, and so if you are interested in your company’s exposure, we do have a report online which you can be able to fill out and request to have sent through to you, which will give you some indications of some of your exposure within the dark web. Then if you find something on there and you’d like to follow up and understand a little bit more, then please get in contact. We’d be happy to take you through, show you around DarkIQ and how that works, and so we’re very much interested in helping you protect your organization’s infrastructure, and we do believe that this outside-in approach is that next-level security that you need on top of your existing security, where you’re doing that inside-out. So, you’re endpoint security, your virus scanners, all those other things that you should be doing, this will help you, even if people have valid credentials to come into your system and your system believes them to be friendly, here’s an extra layer of protection and oversight for you to be able to try and protect yourselves against those threats, who look like they’re friendly, but they’re actually not. Somebody has found a way into your organization through those vital internal security, inside-out looking tools that you have within the system. So, check out our website. Within the website I think there will be a link in the podcast, you can then follow through, run the report on your company, and then follow up with us, and we can talk you through some of those results.
(TC: 00:47:21)
Aidan Murphy: Brilliant, well, that’s a good note to draw a line under this episode of The Dark Dive. A big thank you to Scott and Ben for joining me. If you’d like to find out more about the study, I mean, I’ve said it several times now, but in case you missed it, you can download the report, it’s in the links, in the show notes, and as Ben just mentioned, there is a dark web exposure report that you can run on your own organization. I’ll also put the link to that in the show notes and visit Searchlight Cyber’s website, and you can find it there as well. As ever, please get in touch if you have any questions using the contact details in the show notes, and remember you can find us on Apple Podcasts, Spotify, YouTube, or whatever podcast app you use, for the full backlog of episodes of The Dark Dive. Until next time, stay safe.